Introduction to AI in Product Recommendation
The advent of artificial intelligence (AI) has revolutionized how online businesses operate, particularly in the area of product recommendation. This transformative technology analyzes browsing and purchasing behaviors to offer precise, tailored product suggestions. AI-driven product recommendation systems improve customer satisfaction, enhance sales, and foster loyalty, making them an indispensable tool in modern e-commerce.
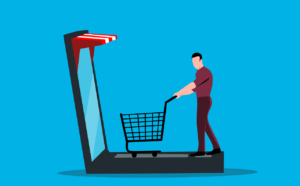
The Impact of AI in Product Recommendation
Enhanced Personalization
AI-powered recommendation systems have transformed the way businesses offer personalized shopping experiences. These advanced systems analyze vast amounts of customer data, including browsing history, past purchases, and search behavior, to predict preferences and recommend relevant products. Unlike traditional recommendation engines that rely on simple algorithms, AI-driven systems utilize sophisticated analytics and machine learning techniques to deliver highly customized suggestions.
Contextual Relevance in Product Recommendations
AI recommendation engines go beyond static data analysis by incorporating context into their predictions. For instance, if a customer searches for winter jackets, AI can analyze real-time factors such as location, weather conditions, and fashion trends to provide the most suitable options. This level of contextual intelligence ensures that recommendations are not only personalized but also highly relevant to the customer’s needs at that moment.
Case Studies: Successful AI Implementations
Amazon’s AI-Driven Recommendation System
Amazon’s recommendation engine is a prime example of AI’s impact on product suggestions. The platform’s “Customers Who Bought This Item Also Bought” feature leverages machine learning to analyze customer purchase histories and behaviors, delivering precise product recommendations. This AI-driven approach has significantly increased Amazon’s sales, customer retention, and engagement.
Netflix and Spotify: AI-Optimized Content Recommendations
Streaming giants like Netflix and Spotify utilize AI to enhance user experience. Netflix’s recommendation system suggests movies and TV shows based on viewing history, ratings, and user interactions. Similarly, Spotify’s AI-driven algorithms curate personalized playlists by analyzing listening habits, ensuring users receive content that aligns with their tastes.
Benefits of AI-Driven Product Recommendations
Boosting Sales and Conversion Rates
AI-powered recommendations contribute to increased sales by showcasing products aligned with customer interests. According to research by Brilliance, personalized recommendations can boost cart additions by 25% and improve purchase completions by 5.5%. AI ensures that customers discover relevant products effortlessly, enhancing overall shopping satisfaction.
Building Customer Loyalty
Personalized recommendations create a sense of individual attention, fostering brand loyalty. Customers are more likely to return to a platform that understands their preferences and provides relevant suggestions. AI-powered personalization strengthens customer relationships and encourages repeat purchases.
Saving Time and Enhancing Convenience
AI streamlines the shopping experience by presenting relevant products, eliminating the need for extensive searching. Customers benefit from a seamless and efficient browsing experience, leading to higher satisfaction and engagement.
Opportunities for Upselling and Cross-Selling
AI identifies opportunities for upselling and cross-selling by recommending premium products or complementary items. For example, if a customer purchases a smartphone, AI can suggest compatible accessories like phone cases and wireless chargers, thereby increasing the average order value and overall revenue.
Key Components
1. Data Collection and Analysis
AI-powered recommendation engines collect and analyze vast amounts of data, including:
- Browsing behavior
- Purchase history
- Demographics
- Customer preferences
Analyzing these data points allows AI to identify patterns and predict customer needs accurately.
2. Machine Learning Algorithms
Machine learning is at the core of AI recommendation systems. These algorithms learn from extensive datasets, identifying trends and behaviors that inform product suggestions. Over time, they refine their recommendations, improving accuracy and relevance.
3. Natural Language Processing (NLP)
NLP enables AI to analyze customer reviews, social media interactions, and feedback to determine popular products and valued features. By understanding customer sentiment, AI can recommend products that align with user preferences and emerging market trends.
4. Collaborative Filtering
Collaborative filtering analyzes customer behaviors and preferences to recommend products that similar customers have purchased. This technique enhances personalization by drawing insights from collective user data, ensuring more precise and tailored suggestions.
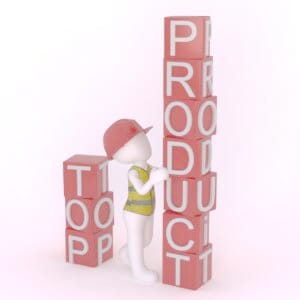
5. Contextual Recommendations
AI-powered recommendation systems provide real-time, contextual suggestions by analyzing customer behavior as it happens. If a user is searching for running shoes, AI can assess factors such as fitness goals, preferred brands, and price range to present the most relevant options.
Future Trends
1. Hyper-Personalization with AI
As AI technology advances, hyper-personalization will become a key differentiator. Businesses will leverage AI to analyze micro-interactions and deliver highly specific product suggestions tailored to each user’s unique preferences.
2. Voice and Visual Search Integration
AI-powered recommendation systems will integrate voice and visual search capabilities. Platforms like Google Lens and Amazon Alexa will enhance product discovery, allowing users to find items through spoken queries or image recognition.
3. AI-Driven Predictive Shopping
Predictive AI will enable ecommerce platforms to anticipate customer needs before they even search for a product. By analyzing past behavior and trends, AI can preemptively recommend products, making shopping more intuitive and effortless.
4. Ethical AI and Data Privacy
As AI in product recommendation evolves, ethical considerations will become increasingly important. Businesses must prioritize data privacy, transparency, and fairness to build trust and maintain compliance with global data protection regulations.
AI in product recommendation is transforming the way businesses interact with customers. Through enhanced personalization, contextual rele
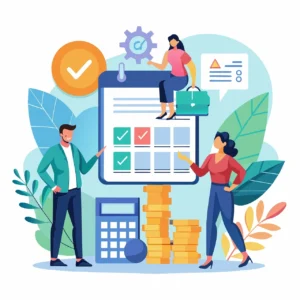
vance, and machine learning-driven insights, AI-powered recommendation systems optimize the shopping experience, drive sales, and foster customer loyalty. Companies that embrace AI-driven product recommendations will gain a competitive edge by delivering tailored, efficient, and engaging experiences that meet the evolving expectations of modern consumers.
Challenges and Ethical Considerations
1. Data Privacy and Security
AI-driven recommendation systems rely on customer data, making privacy protection essential. Businesses must comply with regulations like GDPR and CCPA to maintain customer trust.
2. Competitive Differentiation
With many e-commerce platforms leveraging AI, businesses must develop unique AI-driven personalization strategies to stand out.
Best Practices for Implementing AI in Shopify Stores
Artificial Intelligence (AI) is transforming eCommerce, and Shopify store owners can leverage AI to improve customer experience, boost sales, and optimize store operations. By using AI-driven product recommendations, personalized marketing, and automated customer service, Shopify merchants can enhance engagement and drive revenue.
Below, we’ll explore best practices for integrating AI into Shopify stores for maximum effectiveness.
1. Define Objectives – Set Clear Goals for AI Implementation
Why This Matters
Before implementing AI in your Shopify store, it’s essential to define clear objectives that align with your business goals. AI can serve various purposes, so knowing what you want to achieve will help guide the implementation process.
Common AI Objectives for Shopify Stores:
✅ Increase revenue – AI-driven recommendations can boost conversions and average order value.
✅ Improve user experience – Personalized shopping experiences enhance customer satisfaction.
✅ Reduce cart abandonment – AI-powered retargeting strategies help recover lost sales.
✅ Enhance customer service – Chatbots and AI-driven responses improve support efficiency.
💡 Pro Tip: Use AI tools like Shopify Inbox, Tidio AI, or Gorgias to automate customer interactions and drive more conversions.
2. Choose the Right AI-Driven Recommendation Engine
Why This Matters
Product recommendation engines are one of the most effective AI tools for Shopify stores. They analyze customer behavior and suggest products based on preferences, browsing history, and purchase patterns. Choosing the right AI-powered recommendation engine ensures personalized and accurate suggestions.
Best AI Recommendation Engines for Shopify:
✅ LimeSpot – AI-driven personalization for product suggestions.
✅ Recom.ai – Smart upselling and cross-selling tool.
✅ Wiser AI – Uses machine learning to recommend products based on past user behavior.
💡 Pro Tip: Choose a recommendation engine that supports personalization, A/B testing, and machine learning-based predictions.
3. Collect and Analyze Customer Data for AI Optimization
Why This Matters
AI systems rely on high-quality data to make accurate predictions and provide personalized experiences. The more relevant data AI has, the better its recommendations and decision-making abilities.
Key Data to Collect for AI Optimization:
✅ Browsing history – Tracks user behavior to recommend relevant products.
✅ Purchase history – AI identifies patterns in buying behavior.
✅ Search queries – Understands what customers are looking for.
✅ Cart and checkout behavior – Helps in reducing cart abandonment.
💡 Pro Tip: Use Google Analytics, Shopify Reports, and Facebook Pixel to collect insights and enhance AI recommendations.
4. Maintain Data Accuracy – Keep Customer Data Updated
Why This Matters
AI systems need fresh, updated data to make accurate predictions. Outdated or incorrect data can lead to irrelevant product recommendations, harming the shopping experience.
Best Practices for Maintaining Data Accuracy:
✅ Sync AI with real-time customer data – Ensure AI recommendations update based on the latest actions.
✅ Segment customer profiles – Group users based on preferences, demographics, and behaviors.
✅ Remove outdated information – Filter irrelevant or old data that could impact AI decisions.
💡 Pro Tip: Use AI-powered CRM tools like HubSpot or Klaviyo to keep customer data accurate and optimized.
5. Utilize Various Recommendation Types for Maximum Engagement
Why This Matters
AI can provide different types of recommendations, making the shopping experience more engaging and increasing conversion rates. Offering multiple recommendation types ensures customers find what they need quickly.
Types of AI Recommendations to Use:
✅ Product-based recommendations – “Customers also bought” or “Frequently bought together.”
✅ Category-based recommendations – Suggesting similar products in the same niche.
✅ Cross-sell recommendations – Offering complementary products to increase order value.
✅ Upsell recommendations – Encouraging customers to upgrade to higher-priced items.
💡 Pro Tip: Use LimeSpot or Recom.ai to implement smart AI-driven recommendations in your Shopify store.
6. Optimize Placement of AI Recommendations for Higher Conversions
Why This Matters
Strategic placement of AI-driven recommendations ensures they are seen by customers at the right time and place. AI product suggestions work best when placed on high-traffic pages that influence purchase decisions.
Best Places to Display AI Recommendations:
✅ Homepage – Showcase trending or personalized product recommendations.
✅ Product pages – Cross-sell or upsell similar items.
✅ Cart and checkout page – Encourage add-ons and bundle purchases.
✅ Post-purchase page – Suggest related items for future purchases.
💡 Pro Tip: Use A/B testing to analyze which placement generates the highest conversions.
7. Test and Optimize AI Performance Regularly
Why This Matters
AI performance improves over time, but continuous testing ensures that the recommendation engine is effective. Regular monitoring allows for data-driven optimization to enhance user engagement and revenue.
How to Optimize AI Recommendations:
✅ A/B test different AI models – Compare multiple AI-driven strategies.
✅ Monitor user behavior – Adjust recommendations based on real-time analytics.
✅ Collect customer feedback – Understand how users interact with AI suggestions.
✅ Adjust AI algorithms regularly – Improve AI accuracy based on new trends.
💡 Pro Tip: Shopify apps like Omnisend, Pendo, and Google Optimize help in testing and improving AI-driven recommendations.
Why AI is Essential for Shopify Success
AI-driven strategies help Shopify stores provide a personalized shopping experience, boost customer engagement, and increase revenue. By implementing these best practices, merchants can make the most out of AI technology.
Quick Recap: Best AI Practices for Shopify
✅ Define Objectives – Set clear goals for AI implementation.
✅ Choose the Right AI Tool – Select the best recommendation engine.
✅ Collect and Analyze Data – Ensure AI has quality customer data.
✅ Maintain Data Accuracy – Keep data fresh for better AI results.
✅ Use Various Recommendation Types – Provide personalized suggestions.
✅ Optimize Placement – Display recommendations strategically.
✅ Test and Optimize – Continuously improve AI performance.
Conclusion
AI in product recommendation is transforming e-commerce by providing personalized, data-driven shopping experiences. With enhanced personalization, contextual relevance, and machine learning-driven insights, AI-powered recommendation engines improve customer satisfaction and boost sales. As AI technology advances, businesses must stay ahead by implementing ethical AI practices, maintaining data privacy, and optimizing recommendation strategies for long-term success.
Frequently Asked Questions (FAQs)
- What is AI in product recommendation?
AI in product recommendation refers to using artificial intelligence technologies to analyze customer data and provide tailored product suggestions.
- Why are AI-powered recommendations important?
They enhance personalization, increase sales, save time, and build customer loyalty by delivering relevant product suggestions.
- How does AI improve recommendation accuracy?
AI analyzes large datasets using machine learning, NLP, and collaborative filtering to identify patterns and preferences.
- Are AI-driven recommendation systems expensive?
Costs vary based on the chosen system, but the long-term benefits, including increased sales and customer satisfaction, often outweigh the investment.
- How can I implement AI recommendations in my Shopify store?
Define your goals, choose a suitable recommendation engine, collect and analyze data, ensure data quality, and continuously test and optimize the system.
- What are the ethical considerations in using AI for recommendations?
Protecting customer data and ensuring ethical usage are critical. Businesses must comply with privacy regulations and build customer trust.
By adopting AI-powered product recommendation systems, businesses can offer personalized shopping experiences, boost sales, and gain a competitive edge in the e-commerce market. Strategic implementation, combined with ethical practices, ensures lasting success and customer satisfaction.